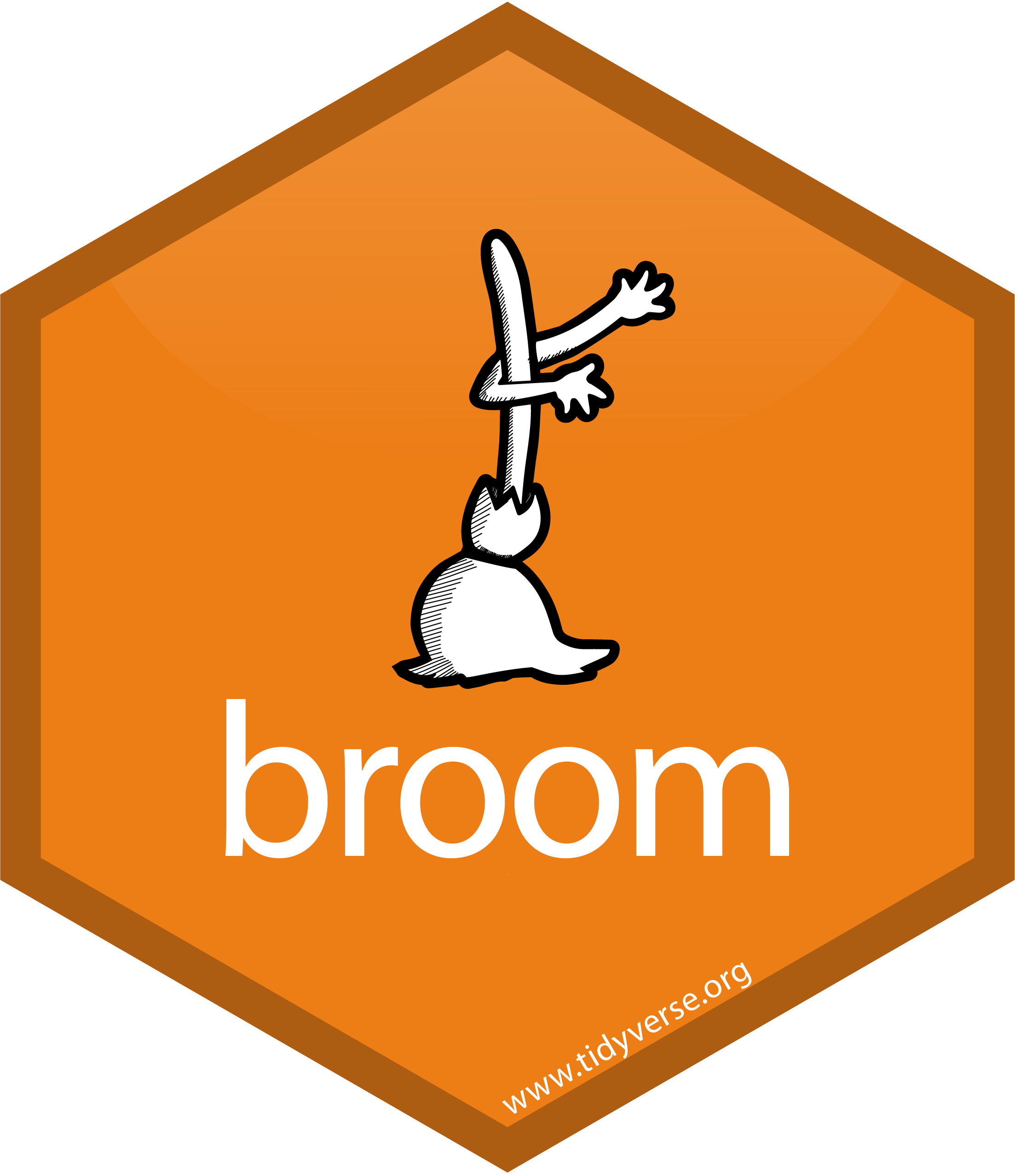
Tidying methods for multinomial logistic regression models
Source:R/nnet-tidiers.R
tidy.multinom.Rd
These methods tidy the coefficients of multinomial logistic regression
models generated by multinom
of the nnet
package.
Usage
# S3 method for class 'multinom'
tidy(x, conf.int = FALSE, conf.level = 0.95, exponentiate = FALSE, ...)
Arguments
- x
A
multinom
object returned fromnnet::multinom()
.- conf.int
Logical indicating whether or not to include a confidence interval in the tidied output. Defaults to
FALSE
.- conf.level
The confidence level to use for the confidence interval if
conf.int = TRUE
. Must be strictly greater than 0 and less than 1. Defaults to 0.95, which corresponds to a 95 percent confidence interval.- exponentiate
Logical indicating whether or not to exponentiate the the coefficient estimates. This is typical for logistic and multinomial regressions, but a bad idea if there is no log or logit link. Defaults to
FALSE
.- ...
Additional arguments. Not used. Needed to match generic signature only. Cautionary note: Misspelled arguments will be absorbed in
...
, where they will be ignored. If the misspelled argument has a default value, the default value will be used. For example, if you passconf.lvel = 0.9
, all computation will proceed usingconf.level = 0.95
. Two exceptions here are:
See also
Other multinom tidiers:
glance.multinom()
Value
A tibble::tibble()
with columns:
- conf.high
Upper bound on the confidence interval for the estimate.
- conf.low
Lower bound on the confidence interval for the estimate.
- estimate
The estimated value of the regression term.
- p.value
The two-sided p-value associated with the observed statistic.
- statistic
The value of a T-statistic to use in a hypothesis that the regression term is non-zero.
- std.error
The standard error of the regression term.
- term
The name of the regression term.
- y.value
The response level.
Examples
# load libraries for models and data
library(nnet)
library(MASS)
example(birthwt)
#>
#> brthwt> bwt <- with(birthwt, {
#> brthwt+ race <- factor(race, labels = c("white", "black", "other"))
#> brthwt+ ptd <- factor(ptl > 0)
#> brthwt+ ftv <- factor(ftv)
#> brthwt+ levels(ftv)[-(1:2)] <- "2+"
#> brthwt+ data.frame(low = factor(low), age, lwt, race, smoke = (smoke > 0),
#> brthwt+ ptd, ht = (ht > 0), ui = (ui > 0), ftv)
#> brthwt+ })
#>
#> brthwt> options(contrasts = c("contr.treatment", "contr.poly"))
#>
#> brthwt> glm(low ~ ., binomial, bwt)
#>
#> Call: glm(formula = low ~ ., family = binomial, data = bwt)
#>
#> Coefficients:
#> (Intercept) age lwt raceblack raceother
#> 0.82302 -0.03723 -0.01565 1.19241 0.74068
#> smokeTRUE ptdTRUE htTRUE uiTRUE ftv1
#> 0.75553 1.34376 1.91317 0.68020 -0.43638
#> ftv2+
#> 0.17901
#>
#> Degrees of Freedom: 188 Total (i.e. Null); 178 Residual
#> Null Deviance: 234.7
#> Residual Deviance: 195.5 AIC: 217.5
bwt.mu <- multinom(low ~ ., bwt)
#> # weights: 12 (11 variable)
#> initial value 131.004817
#> iter 10 value 98.029803
#> final value 97.737759
#> converged
tidy(bwt.mu)
#> # A tibble: 11 × 6
#> y.level term estimate std.error statistic p.value
#> <chr> <chr> <dbl> <dbl> <dbl> <dbl>
#> 1 1 (Intercept) 0.823 1.24 0.661 0.508
#> 2 1 age -0.0372 0.0387 -0.962 0.336
#> 3 1 lwt -0.0157 0.00708 -2.21 0.0271
#> 4 1 raceblack 1.19 0.536 2.22 0.0261
#> 5 1 raceother 0.741 0.462 1.60 0.109
#> 6 1 smokeTRUE 0.756 0.425 1.78 0.0755
#> 7 1 ptdTRUE 1.34 0.481 2.80 0.00518
#> 8 1 htTRUE 1.91 0.721 2.65 0.00794
#> 9 1 uiTRUE 0.680 0.464 1.46 0.143
#> 10 1 ftv1 -0.436 0.479 -0.910 0.363
#> 11 1 ftv2+ 0.179 0.456 0.392 0.695
glance(bwt.mu)
#> # A tibble: 1 × 4
#> edf deviance AIC nobs
#> <dbl> <dbl> <dbl> <int>
#> 1 11 195. 217. 189
# or, for output from a multinomial logistic regression
fit.gear <- multinom(gear ~ mpg + factor(am), data = mtcars)
#> # weights: 12 (6 variable)
#> initial value 35.155593
#> iter 10 value 14.156582
#> iter 20 value 14.031881
#> iter 30 value 14.025659
#> iter 40 value 14.021414
#> iter 50 value 14.019824
#> iter 60 value 14.019278
#> iter 70 value 14.018601
#> iter 80 value 14.018282
#> iter 80 value 14.018282
#> iter 90 value 14.017126
#> final value 14.015374
#> converged
tidy(fit.gear)
#> # A tibble: 6 × 6
#> y.level term estimate std.error statistic p.value
#> <chr> <chr> <dbl> <dbl> <dbl> <dbl>
#> 1 4 (Intercept) -11.2 5.32 -2.10 3.60e- 2
#> 2 4 mpg 0.525 0.268 1.96 5.02e- 2
#> 3 4 factor(am)1 11.9 66.9 0.178 8.59e- 1
#> 4 5 (Intercept) -18.4 67.9 -0.271 7.87e- 1
#> 5 5 mpg 0.366 0.292 1.25 2.10e- 1
#> 6 5 factor(am)1 22.4 2.17 10.3 4.54e-25
glance(fit.gear)
#> # A tibble: 1 × 4
#> edf deviance AIC nobs
#> <dbl> <dbl> <dbl> <int>
#> 1 6 28.0 40.0 32